Written by Rakesh Ranjan, Basavaraj R. Amogi, Lav R. Khot, R. Troy Peters, Center for Precision and Automated Agricultural Systems, Department of Biological Systems Engineering, Washington State University, Prosser, July 2021
Introduction
Sunburn is a major issue in apple production, resulting up to 40% yield loss (Schrader et al., 2006). Sunburn adversely affects the fruit aesthetics and reduces marketability. For several years, the fruit surface temperature (FST) has been used as a reliable indicator of the sunburn susceptibility (Rackso and Schrader, 2012). Several contact type instruments, e.g., thermocouples, thermal probes, etc. have been used to measure FST. However, these methods can be inaccurate, data-limited, destructive, and laborious (Wang et al., 2020). Therefore, our research group has developed and validated a field-deployable, crop physiology sensing system (CPSS) prototype for accurate and real-time apple FST estimation.
System Details
Each CPSS consists of a sensing module, on-the-edge data processing unit, and networking hardware for remote accessibility and data visualization. The sensing module is comprised of a thermal-RGB sensor (model: FLIR Duo R, FLIR Systems, Inc., Wilsonville, OR) and an all-in-one weather sensor (model: ATMOS 41, METER Group, Inc., Pullman, WA). The acquired imagery data is analyzed on-board the 1.2 GHz ARM Cortex–A53 processing unit (Raspberry Pi V3B, Raspberry Pi foundation, Cambridge, UK) and uses an image processing algorithm developed by our research group (Shi et al., 2019). The CPSS also derives FST using in-orchard weather data (solar radiation, wind speed, air temperature, dew point temperature) and fruit attributes (fruit size, emissivity, and albedo) as input to an energy balance model (Li et al., 2014)). Each node is Internet of Things (IoT) enabled and can share the FST estimates (weather & imagery based) to the end user’s personal computer or smartphone devices.
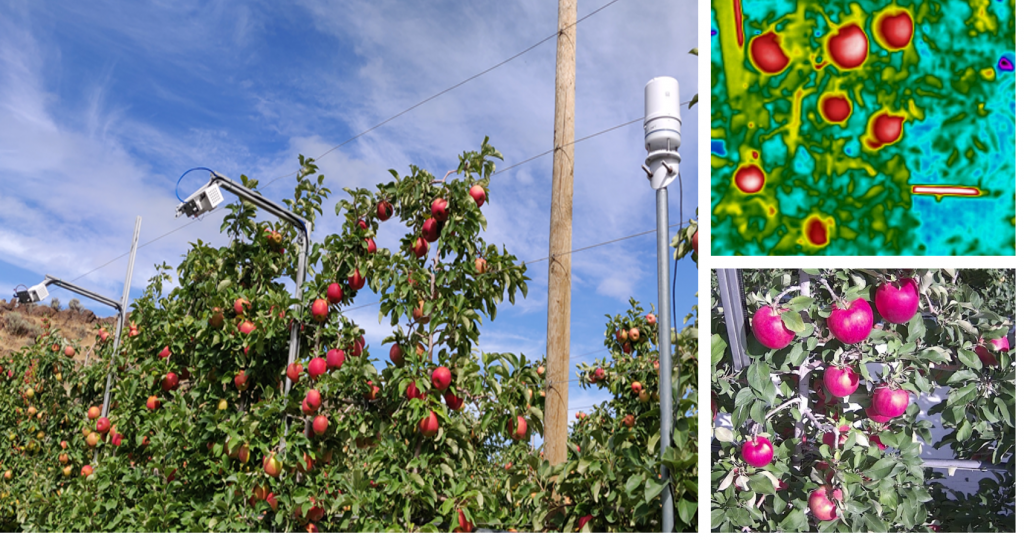
Field validation
Two CPSS nodes were field deployed in the summer of 2019 in a commercial apple orchard (cv. Honeycrisp) and programmed to acquire imagery and in-orchard weather data at an interval of 5 minutes (Figure 1). The imagery data was used to estimate imagery based mean FST and the FST of the hottest 10% of the fruit. A handheld thermal probe (accuracy = ± 0.4℃) was used to assess the actual FST of sun-exposed fruits (Figure 2). The CPSS derived imagery and weather based FST was validated against the actual FST. Additionally, a sensitivity analysis was conducted on the weather data-based energy balance model (Li et al., 2014) to investigate the effect of crop parameters on the model performance. We also conducted a season-long trial in summer of 2020 to validate the CPSS for sunburn and heat stress monitoring in Cosmic Crisp cultivar. More specifics on sensing system and field validation are in Ranjan et al. (2020a,b).
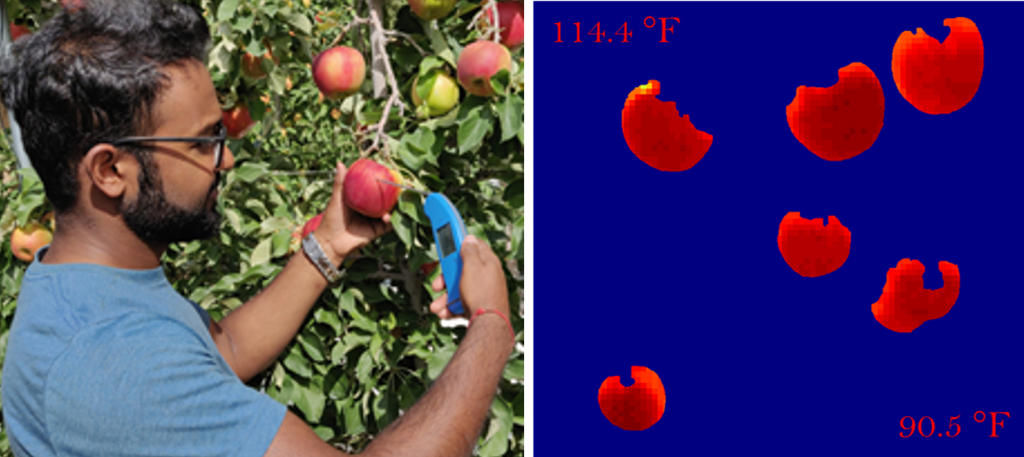
Results and Discussion
Imagery based FST collected in 2019 was strongly correlated with actual FST (R2 = 0.64, p < 0.001) (Figure 3a). Moreover, there was no significant difference in the imagery based FST (mean ± standard error) (100.2 ± 0.4°F) and actual FST (100 ± 0.5°F). During solar noon periods, there was a marginal temperature difference (~1.8 °F) between imagery based mean FST and mean FST of 10% hottest pixels, indicating uniform fruit heating. This difference amplified to ~10.8°F during late afternoon (4:30 pm), indicating localized heating when incident solar rays were perpendicular to the sunlit region (Figure 3b). Also, a temperature difference of 18–27℃ was recorded between air temperature and imagery-based apple FST (Figure 3b). It implies that mean FST or air-temperature alone cannot be reliably utilized for sunburn susceptibility mapping.
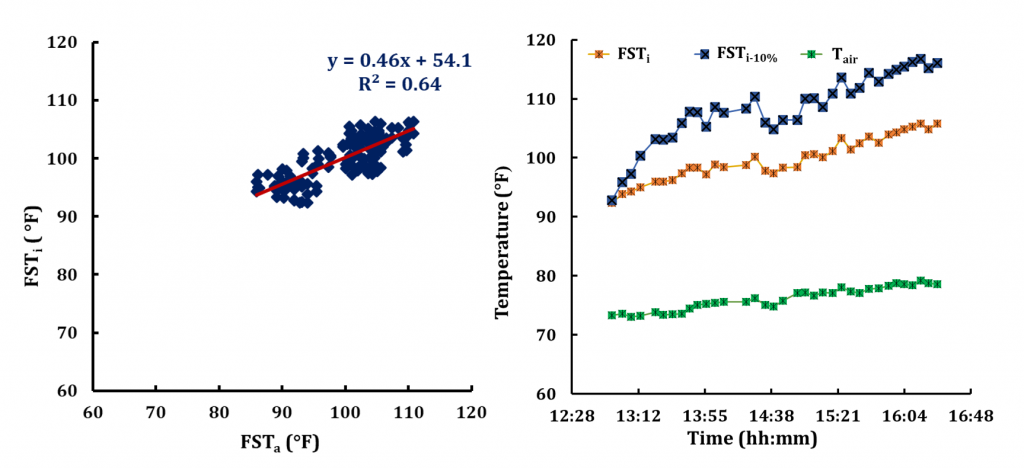
Unlike imagery based mean FST, weather data based FST (108.1 ± 0.7 ℃) was significantly higher than actual FST. Additionally, there was a weaker correlation between weather data based FST estimates and actual FST (R2 = 0.42, p < 0.001). Similar results were obtained from a season-long trial in 2020 for cosmic crisp cultivar. Overall, the thermal RBG imagery data reliably estimated FST and can be used for sunburn management related decision-making. The weather data- based energy balance model depends on several dynamic factors such as fruit shading condition, albedo, emissivity, and fruit diameter. Some of these attributes are cultivar dependent (e.g., albedo and emissivity). We therefore conducted sensitivity analysis of the weather data-based energy balance model. Pertinent results are in Figure 4. Briefly, ±2%, ±100%, ±20%, and ±25% variation in the emissivity, shading condition, albedo and fruit diameter, respectively added an error of 1.6 °F, 14.6 °F, 4.3 °F, and 6.1 °F in FST estimates (Figure 4). The energy balance model also dependents on quality and accuracy of the in-orchard weather data. Thus, sensor selection, installation, and maintenance are critically important for growers to use weather data based FST estimates. Our on-going efforts are focused on collecting cultivar specific fruit parameters as an input to the energy balance model for reliable FST estimation.
Overall, CPSS reliably monitored the heat stress in apples for the 2019 (Honeycrisp) and 2020 (Cosmic Crisp) production season. The imagery based mean FST was found consistent with the actual FST. With further refinement in the weather based FST model parameters, such tools can be reliably adopted for sunburn susceptibility mapping and risk assessment. The real-time FST data stream can be utilized to regulate the evaporative cooling for water and energy efficient sunburn protection and reduced crop losses.
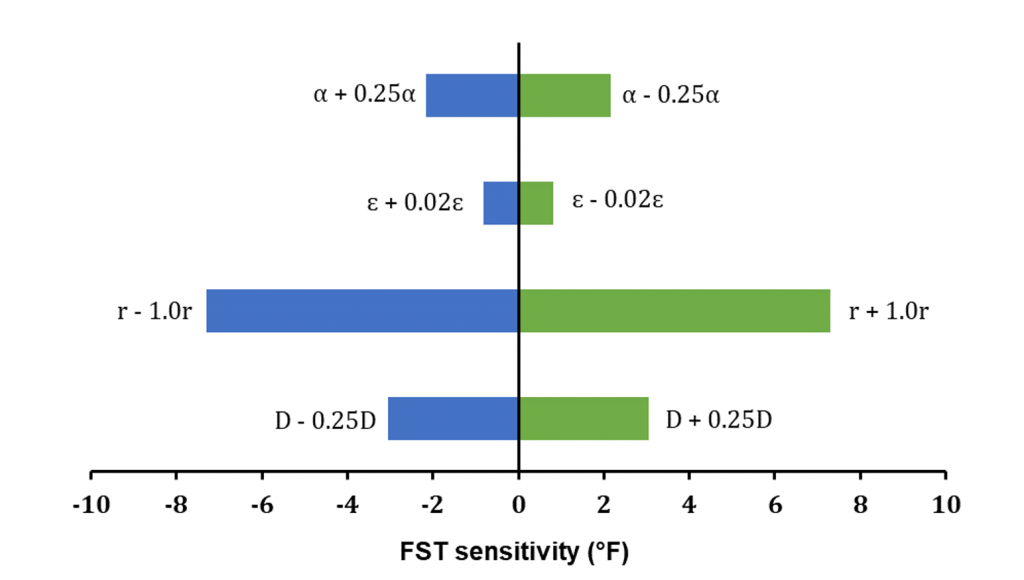
References
Li, L., Peters, T., Zhang, Q., Zhang, J., Huang, D., 2014. Modeling apple surface temperature dynamics based on weather data. Sensors (Basel) 14 (11), 20217–20234.
Ranjan, R., Khot, L. R., Peters, R. T., Salazar-Gutierrez, M. R., Shi, G., 2020a. In-field crop physiology sensing aided real-time apple fruit surface temperature monitoring for sunburn prediction. Comput. Electron. Agric. 175, 105558. doi: https://doi.org/10.1016/j.compag.2020.105558
Ranjan, R., Sinha, R., Khot, L. R., Peters, R. T., Salazar-Gutierrez, M. R. 2020b. Internet of Things enabled crop physiology sensing system for abiotic crop stress management in apple and sweet cherry. In 2020 IEEE International Workshop on Metrology for Agriculture and Forestry. 273–277. doi: https://doi.org/10.1109/MetroAgriFor50201.2020.9277581
Racsko, J., Schrader, L. E., 2012. Sunburn of apple fruit: historical background, recent advances and future perspectives. Crit. Rev. Plant Sci. 31(6), 455–504.
Schrader, L., Sun, J., Zhang, J., Felicetti, D., Tian, J. U. N., 2006. Heat and light-induced apple skin disorders: Causes and prevention. In XXVII International Horticultural Congress-IHC2006: International Symposium on Enhancing Economic and Environmental 772, 51–58.
Shi, G., Ranjan, R., Khot, L.R., 2020. Robust image processing algorithm for computational resource limited smart apple sunburn sensing system. Inf. Process. Agric 7(2), 212–222. doi: https://doi.org/10.1016/j.inpa.2019.09.007.
Wang, B., Ranjan, R., Khot, L. R., Peters, R. T., 2020. Smartphone application-enabled apple fruit surface temperature monitoring tool for in-field and real-time sunburn susceptibility prediction. Sensors (Basel), 20(3), 608. doi: https://doi.org/10.3390/